As a team of students from MIT’s Operations Research, Electrical Engineering & Computer Science, and Leader for Global Operations program, we created the MIMO Student Research Forum to share and discuss how machine intelligence can be applied to solve problems in manufacturing and operations. The Forum is a weekly discussion group organized into topic groups, such as fault detection, robustness, machine vision, and predictive maintenance. Each topic will involve discussions with both industry speakers and MIT student and faculty researchers sharing current methodological and application-oriented work.
These sessions are designed to be more interactive than the typical seminar series, and a substantial portion of the time will be dedicated toward interactive discussion where participants share questions and suggestions. All students interested in machine intelligence methods for application to manufacturing and operations and willing to participate in discussion are welcome to join. Participants are strongly encouraged to present their own work, particularly work that is in process, and suggest topics of interest for future discussion! We hope that through this forum students, faculty, and industry partners can learn about the most critical issues facing operations today and start building the solutions.
All regularly scheduled discussion sessions will be hosted virtually on Thursdays from 5:30-6:30 PM EST. Please join our mailing list to learn more about upcoming events:
http://mailman.mit.edu/mailman/listinfo/mimo-student-forum
Looking forward to seeing you there,
MIMO Student Leadership
MIMO Student Forum, Fall 2020
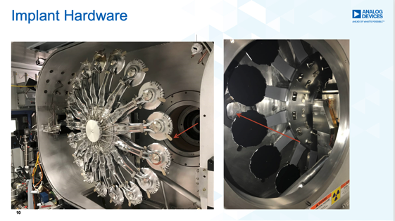
Theme: Fault Detection
Thursday, October 22nd : Jack Dillon, Analog Devices
Faults in semiconductor manufacturing can be extremely costly, ruining entire fabricated wafers that require a significant number of costly fabrication steps in order to create. Quickly detecting these faults is critical to limiting damage to the initial affected batch, preventing subsequent batch failures in the future, and therefore minimizing yield loss. Here, we look at past real-world examples of this phenomenon and discuss how machine learning-based fault detection can limit yield losses in the semiconductor manufacturing industry.
Thursday, October 29th: Chris Lang, MIT

Last week, we heard about the challenges and opportunities that faults can present to the semiconductor manufacturing industry. This week, we take a closer look at methods that can detect faults and can be trained using only known good data. Primarily, we focus on the use of Parzen window classifiers to detect faults in the previously discussed Ion Implantation and Plasma Etch processes. This method is a density estimation technique that can be used to classify new data by evaluating the likelihood that it came from the same distribution as the known good training set. We cover the method general method itself, as well as application-specific decisions, such as its training process, the kernel choice, and adjustments to deal with concept drift.